What if we combine AI tools to assess breast cancer risk?
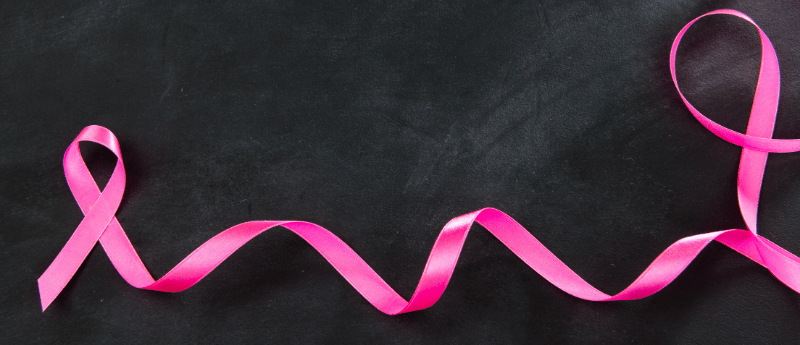
Researchers have taken a strength in numbers approach to breast cancer screening; through combining two diagnostic AI tools they hope to improve overall breast cancer risk assessment.
We’ve all heard of the seemingly endless AI medical diagnostic tools that have been, are currently and will eventually be developed, and most of them carry no particularly groundbreaking results. They all have their disadvantages and limitations, and people are reluctant to trust machine (AI) over man (doctor).
But what if we combine them? There is strength in numbers after all.
That’s exactly what a group of researchers from the Departments of Computer Science and Public Health at the University of Copenhagen (Denmark) attempted to do in a recent study published in Radiology.
According to the World Health Organization, breast cancer affects around 1 in 10 women worldwide, making it the most common cancer in women overall. Early detection and risk assessment is one of the main ways to reduce breast cancer mortality.
Previous research has demonstrated that using mammography-based deep learning models (analyzing lesions) could improve the accuracy of estimating short-term breast cancer risk while texture AI models (analyzing breast density) are more suited for predicting long-term breast cancer risk. No AI models have combined the power of both mammography and texture-based technology.
Until now…
In this study, the team set out to discover if a commercially available AI diagnostic tool (Transpara), in addition to an AI texture model, could be merged to enhance breast cancer risk assessment.
The models were firstly trained separately on a large dataset of almost 40,000 breast cancer exams, and then combined using a deep learning neural network.
To assess the combined strength and accuracy of the two models, the joint force was tested on over 119,000 women who took part in a breast cancer screening program in Denmark between 2012 and 2015. Each model was also tested in isolation using the same dataset.
The model collaboration did indeed improve overall risk assessment for detecting both short-term and long-term breast cancer, achieving a higher area under the receiver operating characteristic curve (AUC) (0.73) when compared to the performance of each individual model (texture risk achieving an AUC of 0.66 and Transpara achieving an AUC of 0.70).
These results could lead to earlier cancer detection and also relieve some of the heavy workload of radiologists worldwide: “Current state-of-the-art clinical risk models require multiple tests such as blood work, genetic testing, mammogram and filling out extensive questionnaires, all of which would substantially increase the workload in the screening clinic,” explained study author Andreas Lauritzen. “Using our model, risk can be assessed with the same performance as the clinical risk models but within seconds from screening and without introducing overhead in the clinic.”