WIRED Health 2025: How Machine Learning is Revolutionizing Drug Discovery
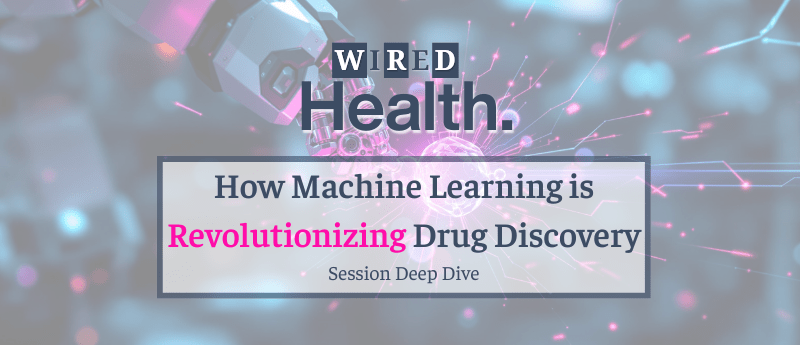
The annual WIRED Health event promises to deliver the most exciting updates to the healthcare world, and this year was no exception. On the 18th of March 2025, leading experts in the field –scientists, doctors, and entrepreneurs alike– came together from across the globe to the city of London (UK) with one goal: to spark discussions on the rapidly changing healthcare landscape.
One of the stand-out talks came from Daphne Koller, Founder and CEO of Insitro. Daphne touched on the transformative potential that machine learning holds for drug discovery, drawing on the recent accomplishments of her own company.
Drug Discovery Inefficiencies: Costing Billions and Wasting Decades
Traditional drug development pipelines are expensive and labor-intensive, typically taking an average of 12 years and $1.3 billion to move a drug from discovery to market. Some studies even suggest this figure stretches as high as $5.5 billion for a single drug.
What’s more, the process is inefficient, with only 10–20% of drugs tested in clinical trials reaching patients. Daphne highlights that a crucial factor behind the low success rate is a fundamental lack of understanding of the biology of different diseases.
“We are going after the wrong targets in the wrong patients. We are basically taking a stab in the dark and then acting surprised when our drugs don’t work.”
Reimagining Drug Discovery with AI
As health technology enters a new era, experts are intrigued by AI’s potential to renovate each step of drug discovery—this is especially true for the initial steps, where automated tools could help researchers rapidly identify therapeutic targets.
Daphne emphasizes the importance of an evidence-based approach in these early stages, using known biological mechanisms of disease as a blueprint for designing new treatments. For example, researchers may find that gene X is frequently mutated in breast cancer patients. Further investigations might reveal that these mutations are linked to uncontrolled cell division and malignancy that lead to breast cancer. This would provide researchers with a basis to create treatments that target this gene.
Crucially, several studies show that using gene-disease associations as a basis for drug development boosts the likelihood of success by 2–3-fold.
Insitro wants to amplify the impact of genetics in drug design, leveraging more data to refine the process. AI is at the heart of this effort, with the company harnessing machine learning (ML) to uncover hidden links between health data and diseases.
Daphne further outlines the potential of AI to fill in missing gaps in datasets, thereby enhancing their quality—a process known as imputation.
“The sad reality of biomedical data is you can never measure everything that you would like to,” she says. “What we need to do is measure what we can and fill in what we cannot, using the power of AI.”
Although imputed data is artificially generated, Daphne stresses that it is anchored in the reality of data from actual humans and cells, much like filling in missing words in a partially completed sentence based on surrounding context.
Insitro in Action: AI-Powered Disease Insights
Insitro is now using imputation to help accelerate drug discovery processes by working hand-in-hand with the pharmaceutical industry. In 2024, the company teamed up with leading company Lilly to support the development of new drugs for metabolic disease.
Under the new partnership, Insitro applied ML to an investigation into the genetic components of fatty liver disease, integrating different types of data from UK Biobank. By analyzing imaging scans, metabolites, and biomarker data, scientists used AI to predict liver fat percentages that are challenging to measure in real time. From here, the researchers could look for new genes associated with elevated liver fat content, exploring new genetic causes of fatty liver disease.
Daphne then goes on to discuss another major area of focus for Insitro: amyotrophic lateral sclerosis (ALS), an incurable neurodegenerative disease that is typically fatal for patients within three to five years of diagnosis.
Insitro, supported by Bristol Myers Squibb, is using AI to investigate the causes of the disease by modeling the effects of different mutations in stem cell-like models.
In one study published in 2024, ML helped researchers analyze images of these cells, extracting subtle features that would have been difficult and time-consuming to pick out manually. They further used ML-based modeling to accurately predict the effect of different mutations commonly seen in the cells of ALS patients.
Daphne hopes that studies such as this will bring researchers closer to developing ALS treatments that improve the quality of patients’ lives or extend life expectancy.
“We’re very excited about a potential disease-modifying treatment for a disease that currently has absolutely nothing available to its patients,” she says.
What’s in Store for Insitro?
Going forward, Insitro is moving full-steam ahead with delivering more AI-powered disease insights.
Just this week, the company announced its latest collaboration with INSIGHT Health Data Research Hub at Moorfields Eye Hospital, London—an NHS-led initiative that collects ocular data for broader health research. Together, the two aim to create a new AI foundational model capable of identifying subtle signs of neurodegeneration from retinal images. This draws inspiration from the emerging field of oculomics, with researchers continually discovering that a host of diseases affect the biological make-up of the eye.
Daphne concludes the talk:
“All of this is driving our work in metabolic, neurologic, and now ophthalmic disease as well, all in service of our mission—to bring better products faster to patients who can benefit the most, through machine learning and data scaling.”