ZleepAnlystNet: A new model for improved sleep stage analysis
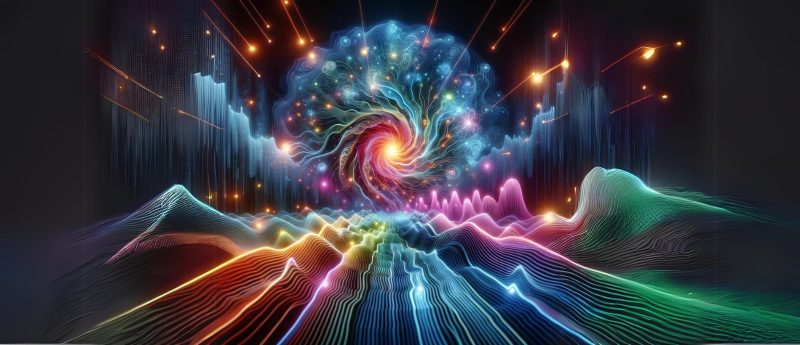
Researchers have developed a novel deep learning model called ZleepAnlystNet, designed to automate sleep stage scoring using single-channel raw electroencephalography (EEG) data.
The approach, detailed in a recent study published in Nature Scientific Reports, introduces a separating training method to enhance model performance distinctly. This method involves separate training for each component of the model, which consists of 15 convolutional neural network models for feature extraction and a single bidirectional long short-term memory model for sequence classification.
The primary strength of ZleepAnlystNet lies in its ability to handle raw EEG data directly, a significant advancement over previous models that often required detailed preprocessing. It achieved an impressive overall accuracy of 87.02%, with a mean F1 score of 82.09% and a Kappa score of 0.8221, indicating a strong agreement with the scores of human sleep technicians.
A key challenge in sleep stage classification has been the accurate identification of the N1 sleep stage, which often shows lower classification performance compared to other stages. The new model addresses this by using class-specific training weights, which help balance the sensitivity across different sleep stages. In testing, the model demonstrated a notable improvement in N1-stage identification.
Unlike traditional end-to-end training, where all parts of the model are trained together, each CNN model is trained separately to optimize its ability to extract relevant features without the interference of downstream classification tasks.
Further, the study also involved cross-dataset validation with the Sleep Heart Health Study dataset, showcasing the model’s adaptability and generalization across different types of EEG data. Although the model’s performance dipped slightly in cross-dataset conditions, it still showed robustness, indicating its potential utility in diverse clinical settings.
This development holds significant promise for enhancing the efficiency of sleep analysis in clinical environments by increasing the throughput of sleep stage scoring in diagnostic settings. Such automated systems can also help standardize sleep stage scoring, reducing variability introduced by human scorers.